A Software Framework for the analysis of time dependent behaviour in production and logistic processes
The use of artificial intelligence has recently been a major driver of innovation within production and logistic processes. Due to this, many methods exist that can be used to analyze process data. However, in practice many of these projects fail, since an elemental information in production and logistic processes – time – is not being considered.
This is despite the fact, that time dependent behavior can show many effects and interdependencies and is usually available without the use of additional sensors. So why is the freely available and invisible sensor time so neglected in the domain of optimization?
The main reason is that durations of time can only be measured between two distinct events – which are usually not defined in production and logistic systems. Measurable events do exist (for example in control signals or state changes of discrete sensors), but usually the events relevant to optimization are hidden in continuous, interdependent and high-dimensional sensor values over time. They are therefore unknown and not explicitly usable for optimization algorithms.
The project Time4CPS therefore aims towards the development of a methodology and a software platform to automatically discretize relevant events from production and logistic processes. These can then be used for system monitoring and optimization.
Research Questions within this project:
RQ1: Discretization and event generation
How can discrete events be extracted from sensor values over time? The input data in this case can be both discrete values as well as continuous values. The learning model must therefore be able to use hybrid data to extract relevant and discrete events from them.
RQ2: Integration of previous knowledge
The use of previous knowledge is usually superior to learning relationships from the process data. Due to this, the use of previous knowledge makes it possible to reduce the amount of needed training data as well as improve the quality of the results. Part of the project will deal with the identification of relevant previous knowledge to support learning and the generation of events.
RQ3: Semantic annotation
The learned events must be available in a standardized data management and be semantically annotated. This information must be defined in the form of ontology models.
RQ4: Optimization of the process flow
The optimization in this project is based on manual adjustments by an expert. To make this possible, a human machine interface needs to be designed, that displays generated events and their context. Therefore, a concept should be developed that outlines the ideal integration of the user into the process.
On the part of the Helmut-Schmidt-University the project is handled by M.Sc. Tom Westermann.
Project duration: 01.07.2020 – 30.06.2022
Project partners
Recogizer Analytics GmbH
ISI Automation GmbH & Co. KG
Hendricks Automotive Group GmbH
GTP Schäfer Gießtechnische Produkte GmbH
Helmut-Schmidt-University
Fraunhofer IOSB-INA
The project Time4CPS is funded by the German ministry of education and research (BMBF) within the research programme Artificial Intelligence in Practice (01IS20002E).
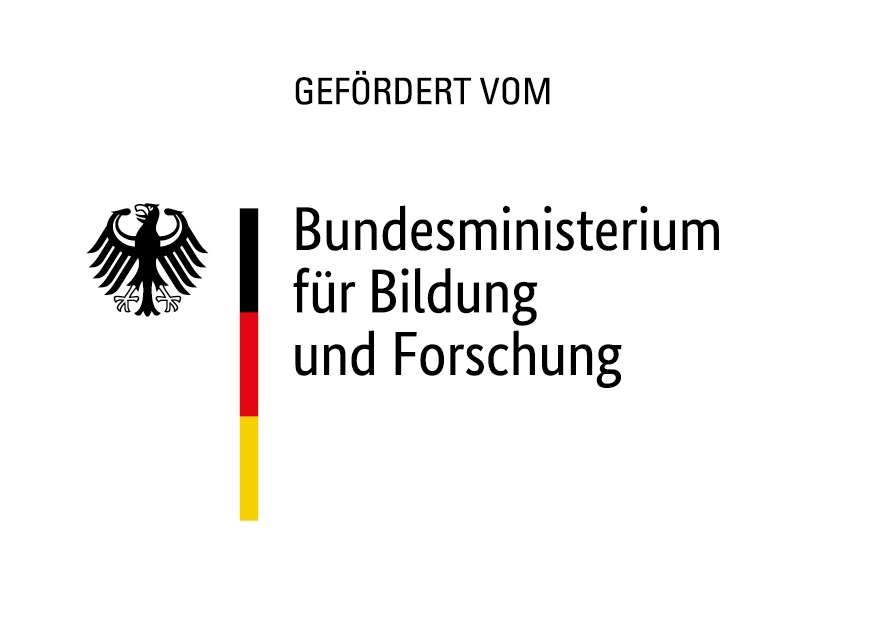
Letzte Änderung: 20. November 2023